Power BI Optimization for a High-Growth SaaS Company
Industry: Information Technology & Services
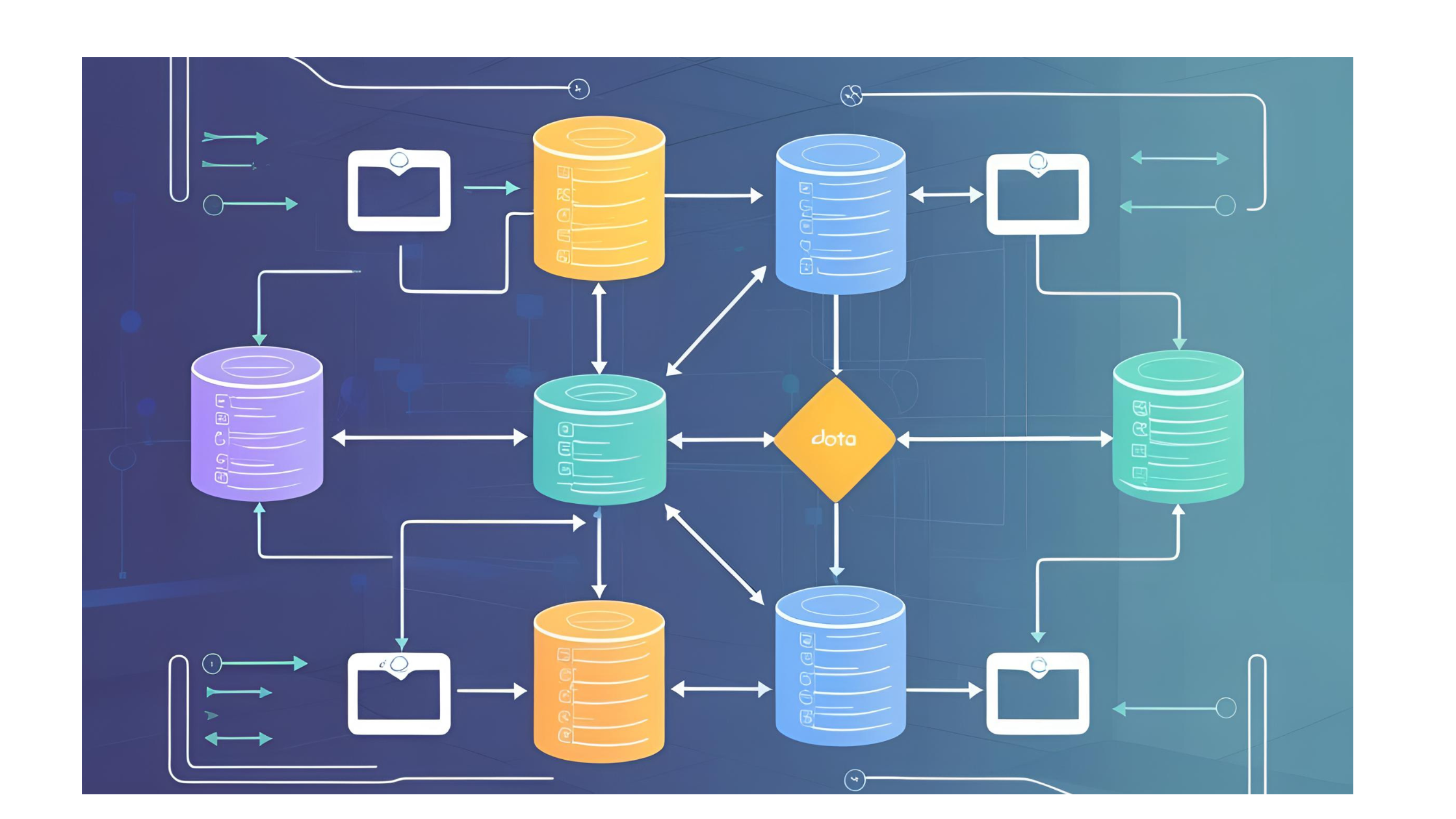
Overview
A fast-growing SaaS company engaged Prexisio to resolve a critical issue in its Power BI environment. Its internal reporting processes had become unsustainable due to excessive and redundant semantic models, which maxed out the service's allocated memory capacity. Prexisio was tasked with identifying the root cause, implementing a scalable solution, and training the internal team for long-term efficiency.
Background
As part of their business intelligence ecosystem, the company heavily relied on Power BI to create dashboards and reports across finance, sales, and operations teams. Their data sources included:
NetSuite for financial and operational data
Salesforce for CRM and pipeline reporting
Adaptive Planning for budgeting and forecasting
Different departments and developers were creating reports independently, without a central governance model for data usage and semantic models.
Challenge
The company hit a critical roadblock: Power BI memory limits were breached, preventing teams from publishing new reports. Each Power BI developer imported the same datasets (e.g., NetSuite or Salesforce) directly into new reports, unintentionally duplicating data and inflating memory usage across dozens of semantic models.
This resulted in:
Redundant data storage
Fragmented business logic
High memory usage in Power BI Service
Inability to deploy new reports and dashboards
No centralized data model strategy led to performance issues, increased cost, and inconsistent reporting logic across departments.
Solution
Prexisio implemented a centralized, scalable semantic model strategy built around performance, governance, and user empowerment.
Key Actions Taken:
Consolidated Semantic Models:
Built three unified models, one each for NetSuite, Salesforce, and Adaptive Planning — each serving as a single source of truth.Streamlined Business Logic:
Preserved and embedded core DAX measures and relationships in the centralized models while allowing developers to add report-specific measures.Data Reduction with Parameters:
Introduced aStartDate
parameter to reduce the data volume ingested into Power BI, optimizing memory usage and speeding up refresh times.Developer Training & Enablement:
Trained Power BI developers to connect to shared semantic models using Power BI Desktop — allowing them to build new reports without re-importing data.
Results
The transformation yielded immediate and measurable benefits:
Reduced semantic models from over 19 to just 3
Resolved memory bottlenecks, allowing reports to be published without delay
Improved consistency across all reports by centralizing business logic
Reduced memory usage and potential overage costs in the Power BI Service
Empowered internal teams with clear best practices and hands-on training for ongoing governance and scalability
Conclusion
By consolidating and optimizing the Power BI environment, Prexisio transformed a chaotic reporting structure into a streamlined, governed, and scalable solution. The SaaS company can now scale reporting without compromising performance, collaboration, or data integrity.
This case highlights Prexisio’s ability to blend technical expertise with business-focused outcomes, ensuring that teams are not only set up for success today, but are also equipped to build smarter and more efficiently moving forward.